Training a classifier when some of the features are unknownWhy does Gradient Boosting regression predict negative values when there are no negative y-values in my training set?How to improve an existing (trained) classifier?What is effect when I set up some self defined predisctor variables?Why Matlab neural network classification returns decimal values on prediction dataset?Fitting and transforming text data in training, testing, and validation setsHow to quantify the performance of the classifier (multi-class SVM) using the test data?How do I control for some patients providing multiple samples in my training data?Training and Test setTraining a convolutional neural network for image denoising in MatlabShouldn't an autoencoder with #(neurons in hidden layer) = #(neurons in input layer) be “perfect”?
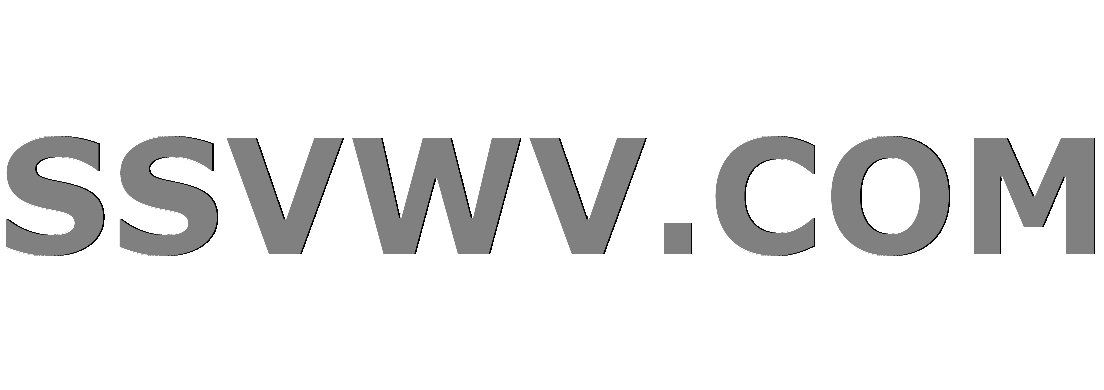
Multi tool use
Extremely casual way to make requests to very close friends
Why are Gatwick's runways too close together?
AsyncDictionary - Can you break thread safety?
Different inverter (logic gate) symbols
Y2K... in 2019?
Withdrew when Jimmy met up with Heath
Christian apologetics regarding the killing of innocent children during the Genesis flood
How does 'AND' distribute over 'OR' (Set Theory)?
Ex-contractor published company source code and secrets online
how to differentiate when a child lwc component is called twice in parent component?
Infeasibility in mathematical optimization models
How is this kind of structure made?
Plausibility of Ice Eaters in the Arctic
Tikzpicture - finish drawing a curved line for a cake slice
Why is transplanting a specific intact brain impossible if it is generally possible?
How to create all combinations from a nested list while preserving the structure using R?
What should I call bands of armed men in Medieval Times?
What happen to those who died but not from the snap?
During the Space Shuttle Columbia Disaster of 2003, Why Did The Flight Director Say, "Lock the doors."?
How can I solve for the intersection points of two ellipses?
Multirow in tabularx?
Can you castle with a "ghost" rook?
changing number of arguments to a function in secondary evaluation
Euler products in positive characteristic? [reference request]
Training a classifier when some of the features are unknown
Why does Gradient Boosting regression predict negative values when there are no negative y-values in my training set?How to improve an existing (trained) classifier?What is effect when I set up some self defined predisctor variables?Why Matlab neural network classification returns decimal values on prediction dataset?Fitting and transforming text data in training, testing, and validation setsHow to quantify the performance of the classifier (multi-class SVM) using the test data?How do I control for some patients providing multiple samples in my training data?Training and Test setTraining a convolutional neural network for image denoising in MatlabShouldn't an autoencoder with #(neurons in hidden layer) = #(neurons in input layer) be “perfect”?
.everyoneloves__top-leaderboard:empty,.everyoneloves__mid-leaderboard:empty,.everyoneloves__bot-mid-leaderboard:empty margin-bottom:0;
$begingroup$
I am training a classifier in Matlab with a dataset that I created.
Unfortunately some of the features in the dataset were not recorded.
I currently have the unknown features set as -99999.
So, for example my dataset looks something like this:
class1: 10 1 12 -99999 6 8
class1: 11 2 13 7 6 10
...
class2: 5 -99999 4 3 2 -99999
class2: -99999 16 4 3 1 8
...
class3: 18 2 11 22 7 5
class3: 19 1 9 25 7 5
...
and so on, where the -99999 are the places where the features werent able to be measured. In this case, each class has 6 features.
I don't want to bias my classifier with the unknown features so I thought it would be a good idea to set the unknowns to -99999 so it would be way out of the range of normal features.
I tested the classifier with the -99999's and it was 78% accurate.
Then I changed the -99999 to 0's and tested the classifier again, this time it was 91% accurate.
So my question is, what is a general rule for training a classifier when some of the features were not recorded? Was I right to assume setting the unknowns to a very high negative value? But why was it more accurate when I set the unknowns to 0s?
Thanks for reading!
machine-learning classification dataset matlab
$endgroup$
add a comment |
$begingroup$
I am training a classifier in Matlab with a dataset that I created.
Unfortunately some of the features in the dataset were not recorded.
I currently have the unknown features set as -99999.
So, for example my dataset looks something like this:
class1: 10 1 12 -99999 6 8
class1: 11 2 13 7 6 10
...
class2: 5 -99999 4 3 2 -99999
class2: -99999 16 4 3 1 8
...
class3: 18 2 11 22 7 5
class3: 19 1 9 25 7 5
...
and so on, where the -99999 are the places where the features werent able to be measured. In this case, each class has 6 features.
I don't want to bias my classifier with the unknown features so I thought it would be a good idea to set the unknowns to -99999 so it would be way out of the range of normal features.
I tested the classifier with the -99999's and it was 78% accurate.
Then I changed the -99999 to 0's and tested the classifier again, this time it was 91% accurate.
So my question is, what is a general rule for training a classifier when some of the features were not recorded? Was I right to assume setting the unknowns to a very high negative value? But why was it more accurate when I set the unknowns to 0s?
Thanks for reading!
machine-learning classification dataset matlab
$endgroup$
add a comment |
$begingroup$
I am training a classifier in Matlab with a dataset that I created.
Unfortunately some of the features in the dataset were not recorded.
I currently have the unknown features set as -99999.
So, for example my dataset looks something like this:
class1: 10 1 12 -99999 6 8
class1: 11 2 13 7 6 10
...
class2: 5 -99999 4 3 2 -99999
class2: -99999 16 4 3 1 8
...
class3: 18 2 11 22 7 5
class3: 19 1 9 25 7 5
...
and so on, where the -99999 are the places where the features werent able to be measured. In this case, each class has 6 features.
I don't want to bias my classifier with the unknown features so I thought it would be a good idea to set the unknowns to -99999 so it would be way out of the range of normal features.
I tested the classifier with the -99999's and it was 78% accurate.
Then I changed the -99999 to 0's and tested the classifier again, this time it was 91% accurate.
So my question is, what is a general rule for training a classifier when some of the features were not recorded? Was I right to assume setting the unknowns to a very high negative value? But why was it more accurate when I set the unknowns to 0s?
Thanks for reading!
machine-learning classification dataset matlab
$endgroup$
I am training a classifier in Matlab with a dataset that I created.
Unfortunately some of the features in the dataset were not recorded.
I currently have the unknown features set as -99999.
So, for example my dataset looks something like this:
class1: 10 1 12 -99999 6 8
class1: 11 2 13 7 6 10
...
class2: 5 -99999 4 3 2 -99999
class2: -99999 16 4 3 1 8
...
class3: 18 2 11 22 7 5
class3: 19 1 9 25 7 5
...
and so on, where the -99999 are the places where the features werent able to be measured. In this case, each class has 6 features.
I don't want to bias my classifier with the unknown features so I thought it would be a good idea to set the unknowns to -99999 so it would be way out of the range of normal features.
I tested the classifier with the -99999's and it was 78% accurate.
Then I changed the -99999 to 0's and tested the classifier again, this time it was 91% accurate.
So my question is, what is a general rule for training a classifier when some of the features were not recorded? Was I right to assume setting the unknowns to a very high negative value? But why was it more accurate when I set the unknowns to 0s?
Thanks for reading!
machine-learning classification dataset matlab
machine-learning classification dataset matlab
edited Apr 15 at 2:46
Darklink9110
asked Apr 15 at 2:40
Darklink9110Darklink9110
235 bronze badges
235 bronze badges
add a comment |
add a comment |
1 Answer
1
active
oldest
votes
$begingroup$
Welcome to Data Science SE!
Well, we say that most of our jobs is to wrangle with data, and that is because data is usually trying to deceive us... jokes aside:
You have a missing data problem
that means your have to clean your data and fill those missing values. To perform this cleaning process your must take the most classic statistician inside of you and ask:
- Why is this data missing?
- How much data is missing?
There are many reasons for a specific information to be unavailable. This will demand you to make assumptions and decide how to deal with this.
Jeff Sauro posted at MeasuringU: 7 Ways to Handle Missing Data, some which I list here:
- Delete corrupted samples:
If you have a large dataset and there is not much data missing, you can simply remove those corrupted data points and go on with life
- Recover the values:
Some problems will allow you to go back and get missing information.
We usually ain't that lucky, then you can
- Educated Guessing:
Sometimes, you can infer what would be the feature value by simply looking their pears. That is a bit arbitrary but it might work.
- Average:
This is the most common approach, simply use the average of that value whenever it is missing. This might artificially reduce your variance but so does using 0 or -9999... for every missing value.
- Regression Substitution:
You can use a multiple regression to infer the missing value from the available values for each candidate.
Some references on missing data are:
- Allison, Paul D. 2001. Missing Data. Sage University Papers
Series on Quantitative Applications in the Social Sciences.
Thousand Oaks: Sage. - Enders, Craig. 2010. Applied Missing Data Analysis.
Guilford Press: New York. - Little, Roderick J., Donald Rubin. 2002. Statistical Analysis
with Missing Data. John Wiley & Sons, Inc: Hoboken. - Schafer, Joseph L., John W. Graham. 2002. “Missing Data:
Our View of the State of the Art.” Psychological Methods.
About your experiment:
Adding -99... is creating outliers and that bit of information is heavy (numerically speaking, it is huge) and will affect parameter tuning. For example, suppose you have this data:
| Feature1 | Feature2 |
|----------|----------|
| 0 | 8 |
| -1 | 7 |
| 1 | - |
| - | 8 |
And you try filling the missing values with -99, now try to fit a linear regression trough the data. Can you see that you don't be able to fit it properly?
The line won't fit, and this will yield bad performance.
Adding 0 values on the other hand will give a slightly better line:
It is still not good, but slightly better since the scale of the parameters will be more realistic.
Now, using average, is this case will give you even better curve, but using regression will give you a perfect fitting line:
Note: I need to remake those images, but these should do until I have the time for it.
$endgroup$
2
$begingroup$
Excellent explanation. Also, just adding to it, I think the best thing to do in data science is to play with missing data. You can (and should) check out Multiple Imputation, this is the state of the art process to deal with missing data.
$endgroup$
– jeff
Apr 15 at 7:20
add a comment |
Your Answer
StackExchange.ready(function()
var channelOptions =
tags: "".split(" "),
id: "557"
;
initTagRenderer("".split(" "), "".split(" "), channelOptions);
StackExchange.using("externalEditor", function()
// Have to fire editor after snippets, if snippets enabled
if (StackExchange.settings.snippets.snippetsEnabled)
StackExchange.using("snippets", function()
createEditor();
);
else
createEditor();
);
function createEditor()
StackExchange.prepareEditor(
heartbeatType: 'answer',
autoActivateHeartbeat: false,
convertImagesToLinks: false,
noModals: true,
showLowRepImageUploadWarning: true,
reputationToPostImages: null,
bindNavPrevention: true,
postfix: "",
imageUploader:
brandingHtml: "Powered by u003ca class="icon-imgur-white" href="https://imgur.com/"u003eu003c/au003e",
contentPolicyHtml: "User contributions licensed under u003ca href="https://creativecommons.org/licenses/by-sa/3.0/"u003ecc by-sa 3.0 with attribution requiredu003c/au003e u003ca href="https://stackoverflow.com/legal/content-policy"u003e(content policy)u003c/au003e",
allowUrls: true
,
onDemand: true,
discardSelector: ".discard-answer"
,immediatelyShowMarkdownHelp:true
);
);
Sign up or log in
StackExchange.ready(function ()
StackExchange.helpers.onClickDraftSave('#login-link');
);
Sign up using Google
Sign up using Facebook
Sign up using Email and Password
Post as a guest
Required, but never shown
StackExchange.ready(
function ()
StackExchange.openid.initPostLogin('.new-post-login', 'https%3a%2f%2fdatascience.stackexchange.com%2fquestions%2f49298%2ftraining-a-classifier-when-some-of-the-features-are-unknown%23new-answer', 'question_page');
);
Post as a guest
Required, but never shown
1 Answer
1
active
oldest
votes
1 Answer
1
active
oldest
votes
active
oldest
votes
active
oldest
votes
$begingroup$
Welcome to Data Science SE!
Well, we say that most of our jobs is to wrangle with data, and that is because data is usually trying to deceive us... jokes aside:
You have a missing data problem
that means your have to clean your data and fill those missing values. To perform this cleaning process your must take the most classic statistician inside of you and ask:
- Why is this data missing?
- How much data is missing?
There are many reasons for a specific information to be unavailable. This will demand you to make assumptions and decide how to deal with this.
Jeff Sauro posted at MeasuringU: 7 Ways to Handle Missing Data, some which I list here:
- Delete corrupted samples:
If you have a large dataset and there is not much data missing, you can simply remove those corrupted data points and go on with life
- Recover the values:
Some problems will allow you to go back and get missing information.
We usually ain't that lucky, then you can
- Educated Guessing:
Sometimes, you can infer what would be the feature value by simply looking their pears. That is a bit arbitrary but it might work.
- Average:
This is the most common approach, simply use the average of that value whenever it is missing. This might artificially reduce your variance but so does using 0 or -9999... for every missing value.
- Regression Substitution:
You can use a multiple regression to infer the missing value from the available values for each candidate.
Some references on missing data are:
- Allison, Paul D. 2001. Missing Data. Sage University Papers
Series on Quantitative Applications in the Social Sciences.
Thousand Oaks: Sage. - Enders, Craig. 2010. Applied Missing Data Analysis.
Guilford Press: New York. - Little, Roderick J., Donald Rubin. 2002. Statistical Analysis
with Missing Data. John Wiley & Sons, Inc: Hoboken. - Schafer, Joseph L., John W. Graham. 2002. “Missing Data:
Our View of the State of the Art.” Psychological Methods.
About your experiment:
Adding -99... is creating outliers and that bit of information is heavy (numerically speaking, it is huge) and will affect parameter tuning. For example, suppose you have this data:
| Feature1 | Feature2 |
|----------|----------|
| 0 | 8 |
| -1 | 7 |
| 1 | - |
| - | 8 |
And you try filling the missing values with -99, now try to fit a linear regression trough the data. Can you see that you don't be able to fit it properly?
The line won't fit, and this will yield bad performance.
Adding 0 values on the other hand will give a slightly better line:
It is still not good, but slightly better since the scale of the parameters will be more realistic.
Now, using average, is this case will give you even better curve, but using regression will give you a perfect fitting line:
Note: I need to remake those images, but these should do until I have the time for it.
$endgroup$
2
$begingroup$
Excellent explanation. Also, just adding to it, I think the best thing to do in data science is to play with missing data. You can (and should) check out Multiple Imputation, this is the state of the art process to deal with missing data.
$endgroup$
– jeff
Apr 15 at 7:20
add a comment |
$begingroup$
Welcome to Data Science SE!
Well, we say that most of our jobs is to wrangle with data, and that is because data is usually trying to deceive us... jokes aside:
You have a missing data problem
that means your have to clean your data and fill those missing values. To perform this cleaning process your must take the most classic statistician inside of you and ask:
- Why is this data missing?
- How much data is missing?
There are many reasons for a specific information to be unavailable. This will demand you to make assumptions and decide how to deal with this.
Jeff Sauro posted at MeasuringU: 7 Ways to Handle Missing Data, some which I list here:
- Delete corrupted samples:
If you have a large dataset and there is not much data missing, you can simply remove those corrupted data points and go on with life
- Recover the values:
Some problems will allow you to go back and get missing information.
We usually ain't that lucky, then you can
- Educated Guessing:
Sometimes, you can infer what would be the feature value by simply looking their pears. That is a bit arbitrary but it might work.
- Average:
This is the most common approach, simply use the average of that value whenever it is missing. This might artificially reduce your variance but so does using 0 or -9999... for every missing value.
- Regression Substitution:
You can use a multiple regression to infer the missing value from the available values for each candidate.
Some references on missing data are:
- Allison, Paul D. 2001. Missing Data. Sage University Papers
Series on Quantitative Applications in the Social Sciences.
Thousand Oaks: Sage. - Enders, Craig. 2010. Applied Missing Data Analysis.
Guilford Press: New York. - Little, Roderick J., Donald Rubin. 2002. Statistical Analysis
with Missing Data. John Wiley & Sons, Inc: Hoboken. - Schafer, Joseph L., John W. Graham. 2002. “Missing Data:
Our View of the State of the Art.” Psychological Methods.
About your experiment:
Adding -99... is creating outliers and that bit of information is heavy (numerically speaking, it is huge) and will affect parameter tuning. For example, suppose you have this data:
| Feature1 | Feature2 |
|----------|----------|
| 0 | 8 |
| -1 | 7 |
| 1 | - |
| - | 8 |
And you try filling the missing values with -99, now try to fit a linear regression trough the data. Can you see that you don't be able to fit it properly?
The line won't fit, and this will yield bad performance.
Adding 0 values on the other hand will give a slightly better line:
It is still not good, but slightly better since the scale of the parameters will be more realistic.
Now, using average, is this case will give you even better curve, but using regression will give you a perfect fitting line:
Note: I need to remake those images, but these should do until I have the time for it.
$endgroup$
2
$begingroup$
Excellent explanation. Also, just adding to it, I think the best thing to do in data science is to play with missing data. You can (and should) check out Multiple Imputation, this is the state of the art process to deal with missing data.
$endgroup$
– jeff
Apr 15 at 7:20
add a comment |
$begingroup$
Welcome to Data Science SE!
Well, we say that most of our jobs is to wrangle with data, and that is because data is usually trying to deceive us... jokes aside:
You have a missing data problem
that means your have to clean your data and fill those missing values. To perform this cleaning process your must take the most classic statistician inside of you and ask:
- Why is this data missing?
- How much data is missing?
There are many reasons for a specific information to be unavailable. This will demand you to make assumptions and decide how to deal with this.
Jeff Sauro posted at MeasuringU: 7 Ways to Handle Missing Data, some which I list here:
- Delete corrupted samples:
If you have a large dataset and there is not much data missing, you can simply remove those corrupted data points and go on with life
- Recover the values:
Some problems will allow you to go back and get missing information.
We usually ain't that lucky, then you can
- Educated Guessing:
Sometimes, you can infer what would be the feature value by simply looking their pears. That is a bit arbitrary but it might work.
- Average:
This is the most common approach, simply use the average of that value whenever it is missing. This might artificially reduce your variance but so does using 0 or -9999... for every missing value.
- Regression Substitution:
You can use a multiple regression to infer the missing value from the available values for each candidate.
Some references on missing data are:
- Allison, Paul D. 2001. Missing Data. Sage University Papers
Series on Quantitative Applications in the Social Sciences.
Thousand Oaks: Sage. - Enders, Craig. 2010. Applied Missing Data Analysis.
Guilford Press: New York. - Little, Roderick J., Donald Rubin. 2002. Statistical Analysis
with Missing Data. John Wiley & Sons, Inc: Hoboken. - Schafer, Joseph L., John W. Graham. 2002. “Missing Data:
Our View of the State of the Art.” Psychological Methods.
About your experiment:
Adding -99... is creating outliers and that bit of information is heavy (numerically speaking, it is huge) and will affect parameter tuning. For example, suppose you have this data:
| Feature1 | Feature2 |
|----------|----------|
| 0 | 8 |
| -1 | 7 |
| 1 | - |
| - | 8 |
And you try filling the missing values with -99, now try to fit a linear regression trough the data. Can you see that you don't be able to fit it properly?
The line won't fit, and this will yield bad performance.
Adding 0 values on the other hand will give a slightly better line:
It is still not good, but slightly better since the scale of the parameters will be more realistic.
Now, using average, is this case will give you even better curve, but using regression will give you a perfect fitting line:
Note: I need to remake those images, but these should do until I have the time for it.
$endgroup$
Welcome to Data Science SE!
Well, we say that most of our jobs is to wrangle with data, and that is because data is usually trying to deceive us... jokes aside:
You have a missing data problem
that means your have to clean your data and fill those missing values. To perform this cleaning process your must take the most classic statistician inside of you and ask:
- Why is this data missing?
- How much data is missing?
There are many reasons for a specific information to be unavailable. This will demand you to make assumptions and decide how to deal with this.
Jeff Sauro posted at MeasuringU: 7 Ways to Handle Missing Data, some which I list here:
- Delete corrupted samples:
If you have a large dataset and there is not much data missing, you can simply remove those corrupted data points and go on with life
- Recover the values:
Some problems will allow you to go back and get missing information.
We usually ain't that lucky, then you can
- Educated Guessing:
Sometimes, you can infer what would be the feature value by simply looking their pears. That is a bit arbitrary but it might work.
- Average:
This is the most common approach, simply use the average of that value whenever it is missing. This might artificially reduce your variance but so does using 0 or -9999... for every missing value.
- Regression Substitution:
You can use a multiple regression to infer the missing value from the available values for each candidate.
Some references on missing data are:
- Allison, Paul D. 2001. Missing Data. Sage University Papers
Series on Quantitative Applications in the Social Sciences.
Thousand Oaks: Sage. - Enders, Craig. 2010. Applied Missing Data Analysis.
Guilford Press: New York. - Little, Roderick J., Donald Rubin. 2002. Statistical Analysis
with Missing Data. John Wiley & Sons, Inc: Hoboken. - Schafer, Joseph L., John W. Graham. 2002. “Missing Data:
Our View of the State of the Art.” Psychological Methods.
About your experiment:
Adding -99... is creating outliers and that bit of information is heavy (numerically speaking, it is huge) and will affect parameter tuning. For example, suppose you have this data:
| Feature1 | Feature2 |
|----------|----------|
| 0 | 8 |
| -1 | 7 |
| 1 | - |
| - | 8 |
And you try filling the missing values with -99, now try to fit a linear regression trough the data. Can you see that you don't be able to fit it properly?
The line won't fit, and this will yield bad performance.
Adding 0 values on the other hand will give a slightly better line:
It is still not good, but slightly better since the scale of the parameters will be more realistic.
Now, using average, is this case will give you even better curve, but using regression will give you a perfect fitting line:
Note: I need to remake those images, but these should do until I have the time for it.
edited Apr 15 at 3:56
answered Apr 15 at 3:30
Pedro Henrique MonfortePedro Henrique Monforte
6892 silver badges19 bronze badges
6892 silver badges19 bronze badges
2
$begingroup$
Excellent explanation. Also, just adding to it, I think the best thing to do in data science is to play with missing data. You can (and should) check out Multiple Imputation, this is the state of the art process to deal with missing data.
$endgroup$
– jeff
Apr 15 at 7:20
add a comment |
2
$begingroup$
Excellent explanation. Also, just adding to it, I think the best thing to do in data science is to play with missing data. You can (and should) check out Multiple Imputation, this is the state of the art process to deal with missing data.
$endgroup$
– jeff
Apr 15 at 7:20
2
2
$begingroup$
Excellent explanation. Also, just adding to it, I think the best thing to do in data science is to play with missing data. You can (and should) check out Multiple Imputation, this is the state of the art process to deal with missing data.
$endgroup$
– jeff
Apr 15 at 7:20
$begingroup$
Excellent explanation. Also, just adding to it, I think the best thing to do in data science is to play with missing data. You can (and should) check out Multiple Imputation, this is the state of the art process to deal with missing data.
$endgroup$
– jeff
Apr 15 at 7:20
add a comment |
Thanks for contributing an answer to Data Science Stack Exchange!
- Please be sure to answer the question. Provide details and share your research!
But avoid …
- Asking for help, clarification, or responding to other answers.
- Making statements based on opinion; back them up with references or personal experience.
Use MathJax to format equations. MathJax reference.
To learn more, see our tips on writing great answers.
Sign up or log in
StackExchange.ready(function ()
StackExchange.helpers.onClickDraftSave('#login-link');
);
Sign up using Google
Sign up using Facebook
Sign up using Email and Password
Post as a guest
Required, but never shown
StackExchange.ready(
function ()
StackExchange.openid.initPostLogin('.new-post-login', 'https%3a%2f%2fdatascience.stackexchange.com%2fquestions%2f49298%2ftraining-a-classifier-when-some-of-the-features-are-unknown%23new-answer', 'question_page');
);
Post as a guest
Required, but never shown
Sign up or log in
StackExchange.ready(function ()
StackExchange.helpers.onClickDraftSave('#login-link');
);
Sign up using Google
Sign up using Facebook
Sign up using Email and Password
Post as a guest
Required, but never shown
Sign up or log in
StackExchange.ready(function ()
StackExchange.helpers.onClickDraftSave('#login-link');
);
Sign up using Google
Sign up using Facebook
Sign up using Email and Password
Post as a guest
Required, but never shown
Sign up or log in
StackExchange.ready(function ()
StackExchange.helpers.onClickDraftSave('#login-link');
);
Sign up using Google
Sign up using Facebook
Sign up using Email and Password
Sign up using Google
Sign up using Facebook
Sign up using Email and Password
Post as a guest
Required, but never shown
Required, but never shown
Required, but never shown
Required, but never shown
Required, but never shown
Required, but never shown
Required, but never shown
Required, but never shown
Required, but never shown
n,37F,L1CVr x3EewOoMl9z9Tmhz5ODaVDK0W6NJKxESu4