Why is the concept of the Null hypothesis associated with the student's t distribution?Is the p-value still uniformly distributed when the null hypothesis is composite?Why use Student's t distribution rather than Student's z distributionHypothesis testing. Why center the sampling distribution on H0?Role of p-value in ruling out null hypothesisWhat is the rationale behind using the t-distribution?Distribution of “p-value-like” quantities under null hypothesisReference for continuous (multivariate) distributions?
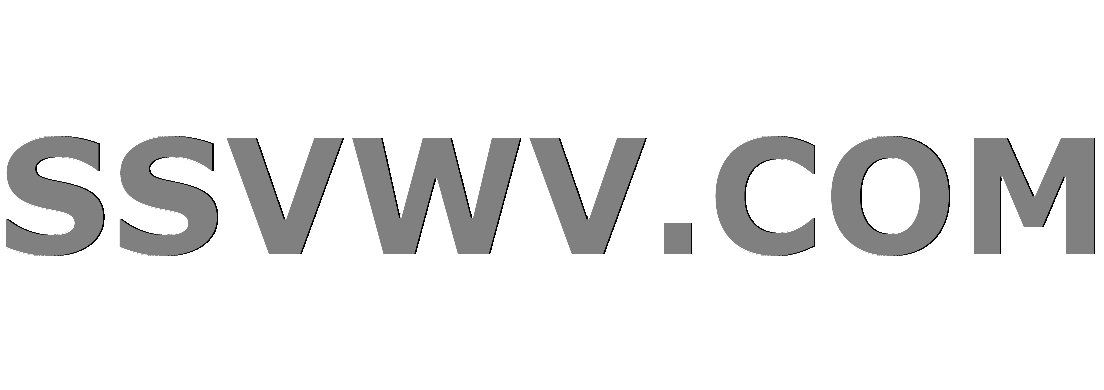
Multi tool use
Right way to say I disagree with the design but ok I will do
Absorption of dark matter by black holes
Is velocity a valid measure of team and process improvement?
Why does Thorin tell Bilbo that he has "keen eyes"?
How is warfare affected when armor has (temporarily) outpaced guns? How can guns compete?
Can someone help explain what this FFT workflow is doing to my signal, and why it works?
Which verb means "to pet <an animal>"?
How offensive is Fachidiot?
Override template and block from a module?
Why is my Windows 7 recovery folder 53% of my disk
How to make a long equation small to fit in LaTeX?
How does an aircraft descend without its nose pointing down?
Colleague used admin access to view my private employee order
Left a meeting without reason, what to do?
Finding big cacti between Phoenix, Las Vegas, and Los Angeles
Can we authenticate external SFTP locations with SSH Key?
Features of a Coda section
Used to Stop a Fight?
QGIS 3.4.6 - symbology - Using Both size and colour to represent two pieces of information on single object
Replacing triangulated categories with something better
The falling broom handle
Are there any real life instances of aircraft aborting a landing to avoid a vehicle?
Using parent's property and will as evidence of assets
Who originated the dangerous avocado-pitting technique?
Why is the concept of the Null hypothesis associated with the student's t distribution?
Is the p-value still uniformly distributed when the null hypothesis is composite?Why use Student's t distribution rather than Student's z distributionHypothesis testing. Why center the sampling distribution on H0?Role of p-value in ruling out null hypothesisWhat is the rationale behind using the t-distribution?Distribution of “p-value-like” quantities under null hypothesisReference for continuous (multivariate) distributions?
.everyoneloves__top-leaderboard:empty,.everyoneloves__mid-leaderboard:empty,.everyoneloves__bot-mid-leaderboard:empty
margin-bottom:0;
$begingroup$
There are dozens of continuous probability distributions like Gaussian (normal), Variance-gamma, Holtsmark, etc. Yet, the concept of the Null hypothesis is basically associated with Student's t-distribution.
Any idea why.
Tanks
probability hypothesis-testing distributions t-test p-value
$endgroup$
add a comment
|
$begingroup$
There are dozens of continuous probability distributions like Gaussian (normal), Variance-gamma, Holtsmark, etc. Yet, the concept of the Null hypothesis is basically associated with Student's t-distribution.
Any idea why.
Tanks
probability hypothesis-testing distributions t-test p-value
$endgroup$
1
$begingroup$
what do you mean by "basically associated?" Are you asking why the t distribution appears to be the most common null distribution in hypothesis testing? The answer might be opinion-based.
$endgroup$
– Taylor
Jun 10 at 23:28
$begingroup$
Yes, I am wondering why t-test is the most used to study the hypothesis test.
$endgroup$
– Ahmed
Jun 10 at 23:52
12
$begingroup$
It isn't. Since hypothesis testing is taught immediately after the t-test in Stats 101, it is usually the first time the "null hypothesis" is taught. And for more than a few people it is also the last time they see it.
$endgroup$
– Peter Leopold
Jun 11 at 2:04
add a comment
|
$begingroup$
There are dozens of continuous probability distributions like Gaussian (normal), Variance-gamma, Holtsmark, etc. Yet, the concept of the Null hypothesis is basically associated with Student's t-distribution.
Any idea why.
Tanks
probability hypothesis-testing distributions t-test p-value
$endgroup$
There are dozens of continuous probability distributions like Gaussian (normal), Variance-gamma, Holtsmark, etc. Yet, the concept of the Null hypothesis is basically associated with Student's t-distribution.
Any idea why.
Tanks
probability hypothesis-testing distributions t-test p-value
probability hypothesis-testing distributions t-test p-value
edited Jun 11 at 13:19
Community♦
1
1
asked Jun 10 at 23:05


AhmedAhmed
256 bronze badges
256 bronze badges
1
$begingroup$
what do you mean by "basically associated?" Are you asking why the t distribution appears to be the most common null distribution in hypothesis testing? The answer might be opinion-based.
$endgroup$
– Taylor
Jun 10 at 23:28
$begingroup$
Yes, I am wondering why t-test is the most used to study the hypothesis test.
$endgroup$
– Ahmed
Jun 10 at 23:52
12
$begingroup$
It isn't. Since hypothesis testing is taught immediately after the t-test in Stats 101, it is usually the first time the "null hypothesis" is taught. And for more than a few people it is also the last time they see it.
$endgroup$
– Peter Leopold
Jun 11 at 2:04
add a comment
|
1
$begingroup$
what do you mean by "basically associated?" Are you asking why the t distribution appears to be the most common null distribution in hypothesis testing? The answer might be opinion-based.
$endgroup$
– Taylor
Jun 10 at 23:28
$begingroup$
Yes, I am wondering why t-test is the most used to study the hypothesis test.
$endgroup$
– Ahmed
Jun 10 at 23:52
12
$begingroup$
It isn't. Since hypothesis testing is taught immediately after the t-test in Stats 101, it is usually the first time the "null hypothesis" is taught. And for more than a few people it is also the last time they see it.
$endgroup$
– Peter Leopold
Jun 11 at 2:04
1
1
$begingroup$
what do you mean by "basically associated?" Are you asking why the t distribution appears to be the most common null distribution in hypothesis testing? The answer might be opinion-based.
$endgroup$
– Taylor
Jun 10 at 23:28
$begingroup$
what do you mean by "basically associated?" Are you asking why the t distribution appears to be the most common null distribution in hypothesis testing? The answer might be opinion-based.
$endgroup$
– Taylor
Jun 10 at 23:28
$begingroup$
Yes, I am wondering why t-test is the most used to study the hypothesis test.
$endgroup$
– Ahmed
Jun 10 at 23:52
$begingroup$
Yes, I am wondering why t-test is the most used to study the hypothesis test.
$endgroup$
– Ahmed
Jun 10 at 23:52
12
12
$begingroup$
It isn't. Since hypothesis testing is taught immediately after the t-test in Stats 101, it is usually the first time the "null hypothesis" is taught. And for more than a few people it is also the last time they see it.
$endgroup$
– Peter Leopold
Jun 11 at 2:04
$begingroup$
It isn't. Since hypothesis testing is taught immediately after the t-test in Stats 101, it is usually the first time the "null hypothesis" is taught. And for more than a few people it is also the last time they see it.
$endgroup$
– Peter Leopold
Jun 11 at 2:04
add a comment
|
4 Answers
4
active
oldest
votes
$begingroup$
There are a dozen of continues probability distributions
There are an infinite number of continuous probability distributions. The ones that have been discussed enough to be named and included in the space of a couple of pages are nevertheless sufficient to fill numerous books (and indeed they do - see, for example, the many books by Johnson, Kotz and other co-authors).
Yet, the concept of the Null hypothesis is basically associated with Student's t-distribution.
This is not the case. If you take a look at either the writing of Neyman and Pearson or that of Fisher on hypothesis testing (the two main approaches to hypothesis testing), the t-distribution is not a necessary nor in any way a major part of either.
Neither is it "the most used to study the hypothesis test" (if you're studying the theory of hypothesis testing you might well only look at it in passing - perhaps as part of one chapter, for example), but it is one of the first examples of hypothesis tests many students learn about.
There are hundreds of hypothesis tests that are used (at least; more probably well into the thousands) and new ones are easy enough to construct. Some situations you may have heard of include: testing independence in contingency tables, testing multinomial goodness of fit, testing equality of means in one way analysis of variance, testing equality of variance, rank based tests of location, or omnibus tests of distributional goodness of fit. None of these are likely to involve t-distributions (and there are many, many more that you probably haven't heard of).
I'd have said the chi-squared distribution and the normal distribution are much more fundamental to hypothesis testing (in particular, as approximations in large samples), but even there, hypothesis tests would still exist even if they didn't come into it at all.
If you look at the Neyman-Pearson lemma, at Fisher exact tests/permutation/randomization testing, and at bootstrap tests, you might instead wonder if the t-distribution would really come up all that much.
Now a substantial subset of tests that are done in applications do involve the t-distribution, but that's in no way an essential property of null hypotheses.
It occurs for a pretty simple reason - it comes up when dealing with inference (tests and intervals) about sample means of normally distributed population quantities (and some other circumstances) under the case where the population variance is unknown.
Consequently the t-distribution (through one-sample/paired t-tests, two sample t-tests, tests of single regression coefficients, and tests of 0 correlation) may be the bulk of your exposure to hypothesis tests but that's not an overwhelming fraction of hypothesis testing more generally.
$endgroup$
add a comment
|
$begingroup$
...the concept of the null hypothesis is basically associated with Student's t-distribution.
Not really. The null hypothesis is associated with a corresponding null distribution, which varies depending on the model and test statistic. In classical hypothesis tests for unknown linear coefficients or mean values, one generally uses a test statistic that is some kind of studentised mean estimator, and this leads to a null distribution which is the Student's T distribution. In other tests, one obtains a different null distribution. It seems that you are associating the two concepts more strongly than they are actually associated, and then wondering why this is.
$endgroup$
add a comment
|
$begingroup$
When we want to test a hypothesis, we need a test statistic with a known probability distribution. This usually involves standardisation of the data. For example, if we collect a random sample $X_1, dots, X_n$ with mean $mu$ and variance $sigma^2$, and the data is assumed to be normally distributed. Then we would standardise it as
$$Z_n = fracbarX_n-musigma/sqrtn$$
$Z_n$ has a standard normal $N(0,1)$ distribution, and so it's values can be used to test whether our hypothesised mean $mu$ is true. Even if the data is not normal, the central limit theorem says that it will be asymptotically provided the variance exists (ie. $EX^2 < infty$).
The problem is that while we are normally interested in the mean $mu$, the variance $sigma^2$ is also unknown. This is called a nuisance parameter. Thus we need to approximate $Z_n$ by substituting in an estimate for $sigma^2$, which is the sample variance
$$s^2 = frac1n-1sum_i=1^n (X_i - barX_n)^2$$
But in doing so we have a new test statistic
$$T_n = fracbarX_n-mu_0s/sqrtn$$
This turns out to have $t$ distribution with $n-1$ degrees of freedom if the null hypothesis is true (ie. if the true mean is used). Thus, even though $sigma^2$ is unknown, we have obtained a test statistic with a well known distribution for which to make inferences.
The reason it follows a $t$ distribution is that the above can be expressed as a normal random variable divided by the square root of an independent chisquared random variable, which gives a $t$ distribution.
$endgroup$
$begingroup$
Thanks for your answer. I am trying to digest the answer, Could you give me a quick example?
$endgroup$
– Ahmed
Jun 10 at 23:53
add a comment
|
$begingroup$
It isn't, but it would probably seem so to a non-statistician who is just learning it while trying to do some basic inference in the context of a science class or something like that. Because the sorts of things in science experiments you want to do inference/hyp testing on have the characteristics of a t-test: the variance is not known, the samples are small, and you are dealing with something that is continuous in nature. A stats student will almost certainly be introduced to a z-test first, through population proportion testing.
The trick is to realize that the transition from z to t test for population mean inference and hypothesis testing comes from the addition of another parameter that needs to be estimated -- the variance -- and in the vast majority of situations you'll encounter the population variance is not known.
I would guess most people who associate hypothesis testing with the T test do so because it's by far the most common one encountered in the sciences and humanities, at least at the lower levels.
$endgroup$
add a comment
|
Your Answer
StackExchange.ready(function()
var channelOptions =
tags: "".split(" "),
id: "65"
;
initTagRenderer("".split(" "), "".split(" "), channelOptions);
StackExchange.using("externalEditor", function()
// Have to fire editor after snippets, if snippets enabled
if (StackExchange.settings.snippets.snippetsEnabled)
StackExchange.using("snippets", function()
createEditor();
);
else
createEditor();
);
function createEditor()
StackExchange.prepareEditor(
heartbeatType: 'answer',
autoActivateHeartbeat: false,
convertImagesToLinks: false,
noModals: true,
showLowRepImageUploadWarning: true,
reputationToPostImages: null,
bindNavPrevention: true,
postfix: "",
imageUploader:
brandingHtml: "Powered by u003ca class="icon-imgur-white" href="https://imgur.com/"u003eu003c/au003e",
contentPolicyHtml: "User contributions licensed under u003ca href="https://creativecommons.org/licenses/by-sa/4.0/"u003ecc by-sa 4.0 with attribution requiredu003c/au003e u003ca href="https://stackoverflow.com/legal/content-policy"u003e(content policy)u003c/au003e",
allowUrls: true
,
onDemand: true,
discardSelector: ".discard-answer"
,immediatelyShowMarkdownHelp:true
);
);
Sign up or log in
StackExchange.ready(function ()
StackExchange.helpers.onClickDraftSave('#login-link');
);
Sign up using Google
Sign up using Facebook
Sign up using Email and Password
Post as a guest
Required, but never shown
StackExchange.ready(
function ()
StackExchange.openid.initPostLogin('.new-post-login', 'https%3a%2f%2fstats.stackexchange.com%2fquestions%2f412393%2fwhy-is-the-concept-of-the-null-hypothesis-associated-with-the-students-t-distri%23new-answer', 'question_page');
);
Post as a guest
Required, but never shown
4 Answers
4
active
oldest
votes
4 Answers
4
active
oldest
votes
active
oldest
votes
active
oldest
votes
$begingroup$
There are a dozen of continues probability distributions
There are an infinite number of continuous probability distributions. The ones that have been discussed enough to be named and included in the space of a couple of pages are nevertheless sufficient to fill numerous books (and indeed they do - see, for example, the many books by Johnson, Kotz and other co-authors).
Yet, the concept of the Null hypothesis is basically associated with Student's t-distribution.
This is not the case. If you take a look at either the writing of Neyman and Pearson or that of Fisher on hypothesis testing (the two main approaches to hypothesis testing), the t-distribution is not a necessary nor in any way a major part of either.
Neither is it "the most used to study the hypothesis test" (if you're studying the theory of hypothesis testing you might well only look at it in passing - perhaps as part of one chapter, for example), but it is one of the first examples of hypothesis tests many students learn about.
There are hundreds of hypothesis tests that are used (at least; more probably well into the thousands) and new ones are easy enough to construct. Some situations you may have heard of include: testing independence in contingency tables, testing multinomial goodness of fit, testing equality of means in one way analysis of variance, testing equality of variance, rank based tests of location, or omnibus tests of distributional goodness of fit. None of these are likely to involve t-distributions (and there are many, many more that you probably haven't heard of).
I'd have said the chi-squared distribution and the normal distribution are much more fundamental to hypothesis testing (in particular, as approximations in large samples), but even there, hypothesis tests would still exist even if they didn't come into it at all.
If you look at the Neyman-Pearson lemma, at Fisher exact tests/permutation/randomization testing, and at bootstrap tests, you might instead wonder if the t-distribution would really come up all that much.
Now a substantial subset of tests that are done in applications do involve the t-distribution, but that's in no way an essential property of null hypotheses.
It occurs for a pretty simple reason - it comes up when dealing with inference (tests and intervals) about sample means of normally distributed population quantities (and some other circumstances) under the case where the population variance is unknown.
Consequently the t-distribution (through one-sample/paired t-tests, two sample t-tests, tests of single regression coefficients, and tests of 0 correlation) may be the bulk of your exposure to hypothesis tests but that's not an overwhelming fraction of hypothesis testing more generally.
$endgroup$
add a comment
|
$begingroup$
There are a dozen of continues probability distributions
There are an infinite number of continuous probability distributions. The ones that have been discussed enough to be named and included in the space of a couple of pages are nevertheless sufficient to fill numerous books (and indeed they do - see, for example, the many books by Johnson, Kotz and other co-authors).
Yet, the concept of the Null hypothesis is basically associated with Student's t-distribution.
This is not the case. If you take a look at either the writing of Neyman and Pearson or that of Fisher on hypothesis testing (the two main approaches to hypothesis testing), the t-distribution is not a necessary nor in any way a major part of either.
Neither is it "the most used to study the hypothesis test" (if you're studying the theory of hypothesis testing you might well only look at it in passing - perhaps as part of one chapter, for example), but it is one of the first examples of hypothesis tests many students learn about.
There are hundreds of hypothesis tests that are used (at least; more probably well into the thousands) and new ones are easy enough to construct. Some situations you may have heard of include: testing independence in contingency tables, testing multinomial goodness of fit, testing equality of means in one way analysis of variance, testing equality of variance, rank based tests of location, or omnibus tests of distributional goodness of fit. None of these are likely to involve t-distributions (and there are many, many more that you probably haven't heard of).
I'd have said the chi-squared distribution and the normal distribution are much more fundamental to hypothesis testing (in particular, as approximations in large samples), but even there, hypothesis tests would still exist even if they didn't come into it at all.
If you look at the Neyman-Pearson lemma, at Fisher exact tests/permutation/randomization testing, and at bootstrap tests, you might instead wonder if the t-distribution would really come up all that much.
Now a substantial subset of tests that are done in applications do involve the t-distribution, but that's in no way an essential property of null hypotheses.
It occurs for a pretty simple reason - it comes up when dealing with inference (tests and intervals) about sample means of normally distributed population quantities (and some other circumstances) under the case where the population variance is unknown.
Consequently the t-distribution (through one-sample/paired t-tests, two sample t-tests, tests of single regression coefficients, and tests of 0 correlation) may be the bulk of your exposure to hypothesis tests but that's not an overwhelming fraction of hypothesis testing more generally.
$endgroup$
add a comment
|
$begingroup$
There are a dozen of continues probability distributions
There are an infinite number of continuous probability distributions. The ones that have been discussed enough to be named and included in the space of a couple of pages are nevertheless sufficient to fill numerous books (and indeed they do - see, for example, the many books by Johnson, Kotz and other co-authors).
Yet, the concept of the Null hypothesis is basically associated with Student's t-distribution.
This is not the case. If you take a look at either the writing of Neyman and Pearson or that of Fisher on hypothesis testing (the two main approaches to hypothesis testing), the t-distribution is not a necessary nor in any way a major part of either.
Neither is it "the most used to study the hypothesis test" (if you're studying the theory of hypothesis testing you might well only look at it in passing - perhaps as part of one chapter, for example), but it is one of the first examples of hypothesis tests many students learn about.
There are hundreds of hypothesis tests that are used (at least; more probably well into the thousands) and new ones are easy enough to construct. Some situations you may have heard of include: testing independence in contingency tables, testing multinomial goodness of fit, testing equality of means in one way analysis of variance, testing equality of variance, rank based tests of location, or omnibus tests of distributional goodness of fit. None of these are likely to involve t-distributions (and there are many, many more that you probably haven't heard of).
I'd have said the chi-squared distribution and the normal distribution are much more fundamental to hypothesis testing (in particular, as approximations in large samples), but even there, hypothesis tests would still exist even if they didn't come into it at all.
If you look at the Neyman-Pearson lemma, at Fisher exact tests/permutation/randomization testing, and at bootstrap tests, you might instead wonder if the t-distribution would really come up all that much.
Now a substantial subset of tests that are done in applications do involve the t-distribution, but that's in no way an essential property of null hypotheses.
It occurs for a pretty simple reason - it comes up when dealing with inference (tests and intervals) about sample means of normally distributed population quantities (and some other circumstances) under the case where the population variance is unknown.
Consequently the t-distribution (through one-sample/paired t-tests, two sample t-tests, tests of single regression coefficients, and tests of 0 correlation) may be the bulk of your exposure to hypothesis tests but that's not an overwhelming fraction of hypothesis testing more generally.
$endgroup$
There are a dozen of continues probability distributions
There are an infinite number of continuous probability distributions. The ones that have been discussed enough to be named and included in the space of a couple of pages are nevertheless sufficient to fill numerous books (and indeed they do - see, for example, the many books by Johnson, Kotz and other co-authors).
Yet, the concept of the Null hypothesis is basically associated with Student's t-distribution.
This is not the case. If you take a look at either the writing of Neyman and Pearson or that of Fisher on hypothesis testing (the two main approaches to hypothesis testing), the t-distribution is not a necessary nor in any way a major part of either.
Neither is it "the most used to study the hypothesis test" (if you're studying the theory of hypothesis testing you might well only look at it in passing - perhaps as part of one chapter, for example), but it is one of the first examples of hypothesis tests many students learn about.
There are hundreds of hypothesis tests that are used (at least; more probably well into the thousands) and new ones are easy enough to construct. Some situations you may have heard of include: testing independence in contingency tables, testing multinomial goodness of fit, testing equality of means in one way analysis of variance, testing equality of variance, rank based tests of location, or omnibus tests of distributional goodness of fit. None of these are likely to involve t-distributions (and there are many, many more that you probably haven't heard of).
I'd have said the chi-squared distribution and the normal distribution are much more fundamental to hypothesis testing (in particular, as approximations in large samples), but even there, hypothesis tests would still exist even if they didn't come into it at all.
If you look at the Neyman-Pearson lemma, at Fisher exact tests/permutation/randomization testing, and at bootstrap tests, you might instead wonder if the t-distribution would really come up all that much.
Now a substantial subset of tests that are done in applications do involve the t-distribution, but that's in no way an essential property of null hypotheses.
It occurs for a pretty simple reason - it comes up when dealing with inference (tests and intervals) about sample means of normally distributed population quantities (and some other circumstances) under the case where the population variance is unknown.
Consequently the t-distribution (through one-sample/paired t-tests, two sample t-tests, tests of single regression coefficients, and tests of 0 correlation) may be the bulk of your exposure to hypothesis tests but that's not an overwhelming fraction of hypothesis testing more generally.
edited Jun 11 at 4:30
answered Jun 11 at 0:43


Glen_bGlen_b
225k23 gold badges449 silver badges801 bronze badges
225k23 gold badges449 silver badges801 bronze badges
add a comment
|
add a comment
|
$begingroup$
...the concept of the null hypothesis is basically associated with Student's t-distribution.
Not really. The null hypothesis is associated with a corresponding null distribution, which varies depending on the model and test statistic. In classical hypothesis tests for unknown linear coefficients or mean values, one generally uses a test statistic that is some kind of studentised mean estimator, and this leads to a null distribution which is the Student's T distribution. In other tests, one obtains a different null distribution. It seems that you are associating the two concepts more strongly than they are actually associated, and then wondering why this is.
$endgroup$
add a comment
|
$begingroup$
...the concept of the null hypothesis is basically associated with Student's t-distribution.
Not really. The null hypothesis is associated with a corresponding null distribution, which varies depending on the model and test statistic. In classical hypothesis tests for unknown linear coefficients or mean values, one generally uses a test statistic that is some kind of studentised mean estimator, and this leads to a null distribution which is the Student's T distribution. In other tests, one obtains a different null distribution. It seems that you are associating the two concepts more strongly than they are actually associated, and then wondering why this is.
$endgroup$
add a comment
|
$begingroup$
...the concept of the null hypothesis is basically associated with Student's t-distribution.
Not really. The null hypothesis is associated with a corresponding null distribution, which varies depending on the model and test statistic. In classical hypothesis tests for unknown linear coefficients or mean values, one generally uses a test statistic that is some kind of studentised mean estimator, and this leads to a null distribution which is the Student's T distribution. In other tests, one obtains a different null distribution. It seems that you are associating the two concepts more strongly than they are actually associated, and then wondering why this is.
$endgroup$
...the concept of the null hypothesis is basically associated with Student's t-distribution.
Not really. The null hypothesis is associated with a corresponding null distribution, which varies depending on the model and test statistic. In classical hypothesis tests for unknown linear coefficients or mean values, one generally uses a test statistic that is some kind of studentised mean estimator, and this leads to a null distribution which is the Student's T distribution. In other tests, one obtains a different null distribution. It seems that you are associating the two concepts more strongly than they are actually associated, and then wondering why this is.
answered Jun 11 at 0:59


BenBen
39.4k2 gold badges51 silver badges170 bronze badges
39.4k2 gold badges51 silver badges170 bronze badges
add a comment
|
add a comment
|
$begingroup$
When we want to test a hypothesis, we need a test statistic with a known probability distribution. This usually involves standardisation of the data. For example, if we collect a random sample $X_1, dots, X_n$ with mean $mu$ and variance $sigma^2$, and the data is assumed to be normally distributed. Then we would standardise it as
$$Z_n = fracbarX_n-musigma/sqrtn$$
$Z_n$ has a standard normal $N(0,1)$ distribution, and so it's values can be used to test whether our hypothesised mean $mu$ is true. Even if the data is not normal, the central limit theorem says that it will be asymptotically provided the variance exists (ie. $EX^2 < infty$).
The problem is that while we are normally interested in the mean $mu$, the variance $sigma^2$ is also unknown. This is called a nuisance parameter. Thus we need to approximate $Z_n$ by substituting in an estimate for $sigma^2$, which is the sample variance
$$s^2 = frac1n-1sum_i=1^n (X_i - barX_n)^2$$
But in doing so we have a new test statistic
$$T_n = fracbarX_n-mu_0s/sqrtn$$
This turns out to have $t$ distribution with $n-1$ degrees of freedom if the null hypothesis is true (ie. if the true mean is used). Thus, even though $sigma^2$ is unknown, we have obtained a test statistic with a well known distribution for which to make inferences.
The reason it follows a $t$ distribution is that the above can be expressed as a normal random variable divided by the square root of an independent chisquared random variable, which gives a $t$ distribution.
$endgroup$
$begingroup$
Thanks for your answer. I am trying to digest the answer, Could you give me a quick example?
$endgroup$
– Ahmed
Jun 10 at 23:53
add a comment
|
$begingroup$
When we want to test a hypothesis, we need a test statistic with a known probability distribution. This usually involves standardisation of the data. For example, if we collect a random sample $X_1, dots, X_n$ with mean $mu$ and variance $sigma^2$, and the data is assumed to be normally distributed. Then we would standardise it as
$$Z_n = fracbarX_n-musigma/sqrtn$$
$Z_n$ has a standard normal $N(0,1)$ distribution, and so it's values can be used to test whether our hypothesised mean $mu$ is true. Even if the data is not normal, the central limit theorem says that it will be asymptotically provided the variance exists (ie. $EX^2 < infty$).
The problem is that while we are normally interested in the mean $mu$, the variance $sigma^2$ is also unknown. This is called a nuisance parameter. Thus we need to approximate $Z_n$ by substituting in an estimate for $sigma^2$, which is the sample variance
$$s^2 = frac1n-1sum_i=1^n (X_i - barX_n)^2$$
But in doing so we have a new test statistic
$$T_n = fracbarX_n-mu_0s/sqrtn$$
This turns out to have $t$ distribution with $n-1$ degrees of freedom if the null hypothesis is true (ie. if the true mean is used). Thus, even though $sigma^2$ is unknown, we have obtained a test statistic with a well known distribution for which to make inferences.
The reason it follows a $t$ distribution is that the above can be expressed as a normal random variable divided by the square root of an independent chisquared random variable, which gives a $t$ distribution.
$endgroup$
$begingroup$
Thanks for your answer. I am trying to digest the answer, Could you give me a quick example?
$endgroup$
– Ahmed
Jun 10 at 23:53
add a comment
|
$begingroup$
When we want to test a hypothesis, we need a test statistic with a known probability distribution. This usually involves standardisation of the data. For example, if we collect a random sample $X_1, dots, X_n$ with mean $mu$ and variance $sigma^2$, and the data is assumed to be normally distributed. Then we would standardise it as
$$Z_n = fracbarX_n-musigma/sqrtn$$
$Z_n$ has a standard normal $N(0,1)$ distribution, and so it's values can be used to test whether our hypothesised mean $mu$ is true. Even if the data is not normal, the central limit theorem says that it will be asymptotically provided the variance exists (ie. $EX^2 < infty$).
The problem is that while we are normally interested in the mean $mu$, the variance $sigma^2$ is also unknown. This is called a nuisance parameter. Thus we need to approximate $Z_n$ by substituting in an estimate for $sigma^2$, which is the sample variance
$$s^2 = frac1n-1sum_i=1^n (X_i - barX_n)^2$$
But in doing so we have a new test statistic
$$T_n = fracbarX_n-mu_0s/sqrtn$$
This turns out to have $t$ distribution with $n-1$ degrees of freedom if the null hypothesis is true (ie. if the true mean is used). Thus, even though $sigma^2$ is unknown, we have obtained a test statistic with a well known distribution for which to make inferences.
The reason it follows a $t$ distribution is that the above can be expressed as a normal random variable divided by the square root of an independent chisquared random variable, which gives a $t$ distribution.
$endgroup$
When we want to test a hypothesis, we need a test statistic with a known probability distribution. This usually involves standardisation of the data. For example, if we collect a random sample $X_1, dots, X_n$ with mean $mu$ and variance $sigma^2$, and the data is assumed to be normally distributed. Then we would standardise it as
$$Z_n = fracbarX_n-musigma/sqrtn$$
$Z_n$ has a standard normal $N(0,1)$ distribution, and so it's values can be used to test whether our hypothesised mean $mu$ is true. Even if the data is not normal, the central limit theorem says that it will be asymptotically provided the variance exists (ie. $EX^2 < infty$).
The problem is that while we are normally interested in the mean $mu$, the variance $sigma^2$ is also unknown. This is called a nuisance parameter. Thus we need to approximate $Z_n$ by substituting in an estimate for $sigma^2$, which is the sample variance
$$s^2 = frac1n-1sum_i=1^n (X_i - barX_n)^2$$
But in doing so we have a new test statistic
$$T_n = fracbarX_n-mu_0s/sqrtn$$
This turns out to have $t$ distribution with $n-1$ degrees of freedom if the null hypothesis is true (ie. if the true mean is used). Thus, even though $sigma^2$ is unknown, we have obtained a test statistic with a well known distribution for which to make inferences.
The reason it follows a $t$ distribution is that the above can be expressed as a normal random variable divided by the square root of an independent chisquared random variable, which gives a $t$ distribution.
answered Jun 10 at 23:20
XiaomiXiaomi
1,3564 silver badges14 bronze badges
1,3564 silver badges14 bronze badges
$begingroup$
Thanks for your answer. I am trying to digest the answer, Could you give me a quick example?
$endgroup$
– Ahmed
Jun 10 at 23:53
add a comment
|
$begingroup$
Thanks for your answer. I am trying to digest the answer, Could you give me a quick example?
$endgroup$
– Ahmed
Jun 10 at 23:53
$begingroup$
Thanks for your answer. I am trying to digest the answer, Could you give me a quick example?
$endgroup$
– Ahmed
Jun 10 at 23:53
$begingroup$
Thanks for your answer. I am trying to digest the answer, Could you give me a quick example?
$endgroup$
– Ahmed
Jun 10 at 23:53
add a comment
|
$begingroup$
It isn't, but it would probably seem so to a non-statistician who is just learning it while trying to do some basic inference in the context of a science class or something like that. Because the sorts of things in science experiments you want to do inference/hyp testing on have the characteristics of a t-test: the variance is not known, the samples are small, and you are dealing with something that is continuous in nature. A stats student will almost certainly be introduced to a z-test first, through population proportion testing.
The trick is to realize that the transition from z to t test for population mean inference and hypothesis testing comes from the addition of another parameter that needs to be estimated -- the variance -- and in the vast majority of situations you'll encounter the population variance is not known.
I would guess most people who associate hypothesis testing with the T test do so because it's by far the most common one encountered in the sciences and humanities, at least at the lower levels.
$endgroup$
add a comment
|
$begingroup$
It isn't, but it would probably seem so to a non-statistician who is just learning it while trying to do some basic inference in the context of a science class or something like that. Because the sorts of things in science experiments you want to do inference/hyp testing on have the characteristics of a t-test: the variance is not known, the samples are small, and you are dealing with something that is continuous in nature. A stats student will almost certainly be introduced to a z-test first, through population proportion testing.
The trick is to realize that the transition from z to t test for population mean inference and hypothesis testing comes from the addition of another parameter that needs to be estimated -- the variance -- and in the vast majority of situations you'll encounter the population variance is not known.
I would guess most people who associate hypothesis testing with the T test do so because it's by far the most common one encountered in the sciences and humanities, at least at the lower levels.
$endgroup$
add a comment
|
$begingroup$
It isn't, but it would probably seem so to a non-statistician who is just learning it while trying to do some basic inference in the context of a science class or something like that. Because the sorts of things in science experiments you want to do inference/hyp testing on have the characteristics of a t-test: the variance is not known, the samples are small, and you are dealing with something that is continuous in nature. A stats student will almost certainly be introduced to a z-test first, through population proportion testing.
The trick is to realize that the transition from z to t test for population mean inference and hypothesis testing comes from the addition of another parameter that needs to be estimated -- the variance -- and in the vast majority of situations you'll encounter the population variance is not known.
I would guess most people who associate hypothesis testing with the T test do so because it's by far the most common one encountered in the sciences and humanities, at least at the lower levels.
$endgroup$
It isn't, but it would probably seem so to a non-statistician who is just learning it while trying to do some basic inference in the context of a science class or something like that. Because the sorts of things in science experiments you want to do inference/hyp testing on have the characteristics of a t-test: the variance is not known, the samples are small, and you are dealing with something that is continuous in nature. A stats student will almost certainly be introduced to a z-test first, through population proportion testing.
The trick is to realize that the transition from z to t test for population mean inference and hypothesis testing comes from the addition of another parameter that needs to be estimated -- the variance -- and in the vast majority of situations you'll encounter the population variance is not known.
I would guess most people who associate hypothesis testing with the T test do so because it's by far the most common one encountered in the sciences and humanities, at least at the lower levels.
answered Jun 11 at 23:25
epseps
194 bronze badges
194 bronze badges
add a comment
|
add a comment
|
Thanks for contributing an answer to Cross Validated!
- Please be sure to answer the question. Provide details and share your research!
But avoid …
- Asking for help, clarification, or responding to other answers.
- Making statements based on opinion; back them up with references or personal experience.
Use MathJax to format equations. MathJax reference.
To learn more, see our tips on writing great answers.
Sign up or log in
StackExchange.ready(function ()
StackExchange.helpers.onClickDraftSave('#login-link');
);
Sign up using Google
Sign up using Facebook
Sign up using Email and Password
Post as a guest
Required, but never shown
StackExchange.ready(
function ()
StackExchange.openid.initPostLogin('.new-post-login', 'https%3a%2f%2fstats.stackexchange.com%2fquestions%2f412393%2fwhy-is-the-concept-of-the-null-hypothesis-associated-with-the-students-t-distri%23new-answer', 'question_page');
);
Post as a guest
Required, but never shown
Sign up or log in
StackExchange.ready(function ()
StackExchange.helpers.onClickDraftSave('#login-link');
);
Sign up using Google
Sign up using Facebook
Sign up using Email and Password
Post as a guest
Required, but never shown
Sign up or log in
StackExchange.ready(function ()
StackExchange.helpers.onClickDraftSave('#login-link');
);
Sign up using Google
Sign up using Facebook
Sign up using Email and Password
Post as a guest
Required, but never shown
Sign up or log in
StackExchange.ready(function ()
StackExchange.helpers.onClickDraftSave('#login-link');
);
Sign up using Google
Sign up using Facebook
Sign up using Email and Password
Sign up using Google
Sign up using Facebook
Sign up using Email and Password
Post as a guest
Required, but never shown
Required, but never shown
Required, but never shown
Required, but never shown
Required, but never shown
Required, but never shown
Required, but never shown
Required, but never shown
Required, but never shown
GT9GNKLz1VkHxFs1hAzkuE d,KGW
1
$begingroup$
what do you mean by "basically associated?" Are you asking why the t distribution appears to be the most common null distribution in hypothesis testing? The answer might be opinion-based.
$endgroup$
– Taylor
Jun 10 at 23:28
$begingroup$
Yes, I am wondering why t-test is the most used to study the hypothesis test.
$endgroup$
– Ahmed
Jun 10 at 23:52
12
$begingroup$
It isn't. Since hypothesis testing is taught immediately after the t-test in Stats 101, it is usually the first time the "null hypothesis" is taught. And for more than a few people it is also the last time they see it.
$endgroup$
– Peter Leopold
Jun 11 at 2:04