What is joint estimation?Variance-gamma distribution: parameter estimationWhat does a “closed-form solution” mean?Can someone explain the concept of nontrivial joint sufficient statistic for a pair of parameters?What does it mean for a sample size to be “too low”, in terms of estimation vs inferrence?Logistic regression diagnostic plots in Rwhat are joint observations?
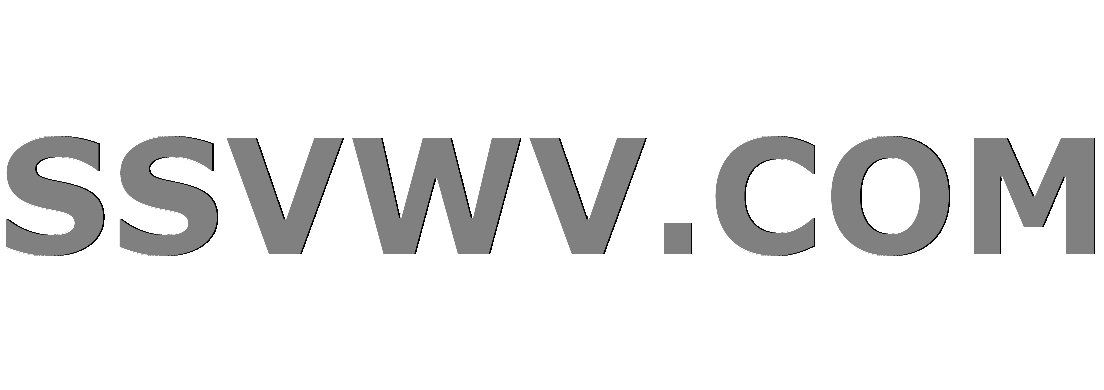
Multi tool use
How to be ready for The Rise of Skywalker?
Lock screen if I leave my seat?
Would the moon eventually fall into Earth if we reduce its tangential velocity slightly?
How to Quickly Replace a Parameter in a Piped Command Chain
How would sword design change if the aim was to cause as much immediate bleeding as possible?
Can every manifold be turned into a Lie group?
what is the essential difference between human languages to other earthly animalia languages?
Magento 2 Escrow Payment Integration
Resolution of potentiometer
How much would a language change over 500 years completely cut off from its source?
Creating 123456 in the fewest number of steps
What sort of professional boundary should I set with other students when I am a grader for a class I will be taking?
Can I rescind my offer of working on weekends after last day?
Implementation gap in logistics
Corridor seats on the TGV — why? Are seat reservations not always mandatory?
Understanding of big-O massively improved when I began thinking of orders as sets. How to apply the same approach to big-Theta?
What game has this black main menu?
A short story (possibility written in the 80's), where humans visit an alien race that evolves fast?
Fill the Golfeek gutter
Which font's digits resemble Nixie tube digits?
Fill your bucket with 2020
Is it possible to conserve the total kinetic energy of a system, but not its momentum?
What does it mean to play "positionally", and how do you train that?
Undercooked areas in chicken breast even though thermometer reads 165 degrees in thickest part
What is joint estimation?
Variance-gamma distribution: parameter estimationWhat does a “closed-form solution” mean?Can someone explain the concept of nontrivial joint sufficient statistic for a pair of parameters?What does it mean for a sample size to be “too low”, in terms of estimation vs inferrence?Logistic regression diagnostic plots in Rwhat are joint observations?
.everyoneloves__top-leaderboard:empty,.everyoneloves__mid-leaderboard:empty,.everyoneloves__bot-mid-leaderboard:empty
margin-bottom:0;
$begingroup$
My question is simple as that: what is joint estimation? And what does it mean in the context of regression analysis? How is it done? I wandered in the mighty Internet for quite some time but did not find answers to these questions.
regression estimation terminology
$endgroup$
add a comment
|
$begingroup$
My question is simple as that: what is joint estimation? And what does it mean in the context of regression analysis? How is it done? I wandered in the mighty Internet for quite some time but did not find answers to these questions.
regression estimation terminology
$endgroup$
1
$begingroup$
Thank you very much for all the helpful answers and the effort you put to clarifying this to me!
$endgroup$
– Lost in regression
Oct 3 at 15:16
add a comment
|
$begingroup$
My question is simple as that: what is joint estimation? And what does it mean in the context of regression analysis? How is it done? I wandered in the mighty Internet for quite some time but did not find answers to these questions.
regression estimation terminology
$endgroup$
My question is simple as that: what is joint estimation? And what does it mean in the context of regression analysis? How is it done? I wandered in the mighty Internet for quite some time but did not find answers to these questions.
regression estimation terminology
regression estimation terminology
edited Oct 3 at 14:19
Richard Hardy
35k7 gold badges55 silver badges156 bronze badges
35k7 gold badges55 silver badges156 bronze badges
asked Oct 2 at 17:20
Lost in regressionLost in regression
1214 bronze badges
1214 bronze badges
1
$begingroup$
Thank you very much for all the helpful answers and the effort you put to clarifying this to me!
$endgroup$
– Lost in regression
Oct 3 at 15:16
add a comment
|
1
$begingroup$
Thank you very much for all the helpful answers and the effort you put to clarifying this to me!
$endgroup$
– Lost in regression
Oct 3 at 15:16
1
1
$begingroup$
Thank you very much for all the helpful answers and the effort you put to clarifying this to me!
$endgroup$
– Lost in regression
Oct 3 at 15:16
$begingroup$
Thank you very much for all the helpful answers and the effort you put to clarifying this to me!
$endgroup$
– Lost in regression
Oct 3 at 15:16
add a comment
|
3 Answers
3
active
oldest
votes
$begingroup$
Joint estimation is, simply, jointly estimating two (or more) things at the same time. It can be as simple as estimating the mean and standard deviation from a sample.
In a lot of the literature, the term is invoked because a special estimating procedure has to be used. This is usually the case when one quantity depends on the other and vice versa so that an analytic solution to the problem is intractable. How exactly joint estimation is done depends entirely on the problem.
One method that pops up often for "joint modeling" or joint estimation is the EM-algorithm. EM stands for expectation - maximization. By alternating these steps, the E-step fills in the missing data that otherwise depend on component A, and the M-step finds optimal estimates for component B. By iterating the E and M steps, you can find a maximum likelihood estimate of A and B, thus jointly estimate these things.
$endgroup$
1
$begingroup$
Can you give an example of where we're not estimating the mean and standard deviation of one variable? What sort of algorithm is used then?
$endgroup$
– smci
Oct 3 at 17:29
$begingroup$
@smci linear mixed modeling jointly estimates the fixed and random components.
$endgroup$
– AdamO
Oct 4 at 21:59
$begingroup$
thanks, I mean, please edit that (and any other examples) into your answer. Is the algorithm totally different to EM? (How does it manage to estimate both components simultaneously? Does it guarantee convergence? etc.)
$endgroup$
– smci
Oct 4 at 22:10
$begingroup$
@smci I disagree. a) That's not the OP's question. b) There are infinite "other examples" c) what is LME's algorithm and how is it different from EM is another question.
$endgroup$
– AdamO
Oct 7 at 15:32
$begingroup$
it helps to illustrate the answer with examples. And that makes for a betetr answer, hence is part of what was asked.
$endgroup$
– smci
Oct 7 at 21:30
add a comment
|
$begingroup$
In a statistical context, the term "joint estimation" could conceivably mean one of two things:
- The simultaneous estimation of two or more scalar parameters (or equivalently, the estimation of a vector parameter with at least two elements); or
- The estimation of a single parameter pertaining to a joint (e.g., in the study of carpentry, plumbing systems, or marijuana smoking).
Of those two options, the second one is a joke, so almost certainly, joint estimation refers to simultaneously estimating two scalar parameters at once.
$endgroup$
1
$begingroup$
to be pedantic, two or more
$endgroup$
– qwr
Oct 3 at 18:23
1
$begingroup$
Pedantry accepted - edited.
$endgroup$
– Reinstate Monica
Oct 3 at 22:20
add a comment
|
$begingroup$
Joint estimation is using data to estimate two or more parameters at the same time. Separate estimation evaluates each parameter one at a time.
Estimation is the result of some form of optimization process. Because of this, there do not exist unique estimation solutions in statistics. If you change your goal, then you change what is optimal. When you first learn things such as regression, no one tells you why you are doing what you are doing. The goal of the instructor is to give you a degree of basic functionality using methods that work in a wide range of circumstances. At the beginning, you are not learning about regression. Instead, you are learning one or two regression methods that are widely applicable in a wide range of circumstances.
The fact you are looking for solutions that solve a hidden goal makes it a bit difficult to understand.
In the context of regression, imagine the following algebraic expression is true $$z=beta_xx+beta_yy+alpha$$. A truism in statistics is the more information that you have, the better off you are. Let us assume that you need to determine what values for $z$ will happen when you see $(x,y)$. The problem is that you do not know the true values for $beta_x,beta_y,alpha$. You have a large, complete data set of $x,y,z$.
In separate estimation, you would estimate one parameter at a time. In joint estimation, you would estimate all of them at once.
As a rule of thumb, joint estimation is more accurate than a separate estimate with a large complete data set. There is one general exception to that. Imagine you have a large set of $x$ and $z$ but a small set of $y$. Imagine most of your $y$ values are missing.
In many estimation routines, you would delete the missing $x$s and $z$s and reduce down the set you are working from until all sets are complete. If you have deleted enough data, it can be more accurate to use the large number of $x$s and $z$s separately to estimate $z=beta_xx+alpha$ and $z=beta_yy+alpha$ than together.
Now as to how it is done. All estimation, excluding a few exceptional cases, uses calculus to find an estimator that minimizes some form of loss or some type of risk. The concern is that you will be unlucky in choosing your sample. Unfortunately, there is an infinite number of loss functions. There is also an infinite number of risk functions.
I found several videos for you because it is a giant topic so that you can look at it in a more general form. They are from Mathematical Monk.
https://www.youtube.com/watch?v=6GhSiM0frIk
https://www.youtube.com/watch?v=5SPm4TmYTX0
https://www.youtube.com/watch?v=b1GxZdFN6cY
and
https://www.youtube.com/watch?v=WdnP1gmb8Hw.
$endgroup$
add a comment
|
Your Answer
StackExchange.ready(function()
var channelOptions =
tags: "".split(" "),
id: "65"
;
initTagRenderer("".split(" "), "".split(" "), channelOptions);
StackExchange.using("externalEditor", function()
// Have to fire editor after snippets, if snippets enabled
if (StackExchange.settings.snippets.snippetsEnabled)
StackExchange.using("snippets", function()
createEditor();
);
else
createEditor();
);
function createEditor()
StackExchange.prepareEditor(
heartbeatType: 'answer',
autoActivateHeartbeat: false,
convertImagesToLinks: false,
noModals: true,
showLowRepImageUploadWarning: true,
reputationToPostImages: null,
bindNavPrevention: true,
postfix: "",
imageUploader:
brandingHtml: "Powered by u003ca class="icon-imgur-white" href="https://imgur.com/"u003eu003c/au003e",
contentPolicyHtml: "User contributions licensed under u003ca href="https://creativecommons.org/licenses/by-sa/4.0/"u003ecc by-sa 4.0 with attribution requiredu003c/au003e u003ca href="https://stackoverflow.com/legal/content-policy"u003e(content policy)u003c/au003e",
allowUrls: true
,
onDemand: true,
discardSelector: ".discard-answer"
,immediatelyShowMarkdownHelp:true
);
);
Sign up or log in
StackExchange.ready(function ()
StackExchange.helpers.onClickDraftSave('#login-link');
);
Sign up using Google
Sign up using Facebook
Sign up using Email and Password
Post as a guest
Required, but never shown
StackExchange.ready(
function ()
StackExchange.openid.initPostLogin('.new-post-login', 'https%3a%2f%2fstats.stackexchange.com%2fquestions%2f429712%2fwhat-is-joint-estimation%23new-answer', 'question_page');
);
Post as a guest
Required, but never shown
3 Answers
3
active
oldest
votes
3 Answers
3
active
oldest
votes
active
oldest
votes
active
oldest
votes
$begingroup$
Joint estimation is, simply, jointly estimating two (or more) things at the same time. It can be as simple as estimating the mean and standard deviation from a sample.
In a lot of the literature, the term is invoked because a special estimating procedure has to be used. This is usually the case when one quantity depends on the other and vice versa so that an analytic solution to the problem is intractable. How exactly joint estimation is done depends entirely on the problem.
One method that pops up often for "joint modeling" or joint estimation is the EM-algorithm. EM stands for expectation - maximization. By alternating these steps, the E-step fills in the missing data that otherwise depend on component A, and the M-step finds optimal estimates for component B. By iterating the E and M steps, you can find a maximum likelihood estimate of A and B, thus jointly estimate these things.
$endgroup$
1
$begingroup$
Can you give an example of where we're not estimating the mean and standard deviation of one variable? What sort of algorithm is used then?
$endgroup$
– smci
Oct 3 at 17:29
$begingroup$
@smci linear mixed modeling jointly estimates the fixed and random components.
$endgroup$
– AdamO
Oct 4 at 21:59
$begingroup$
thanks, I mean, please edit that (and any other examples) into your answer. Is the algorithm totally different to EM? (How does it manage to estimate both components simultaneously? Does it guarantee convergence? etc.)
$endgroup$
– smci
Oct 4 at 22:10
$begingroup$
@smci I disagree. a) That's not the OP's question. b) There are infinite "other examples" c) what is LME's algorithm and how is it different from EM is another question.
$endgroup$
– AdamO
Oct 7 at 15:32
$begingroup$
it helps to illustrate the answer with examples. And that makes for a betetr answer, hence is part of what was asked.
$endgroup$
– smci
Oct 7 at 21:30
add a comment
|
$begingroup$
Joint estimation is, simply, jointly estimating two (or more) things at the same time. It can be as simple as estimating the mean and standard deviation from a sample.
In a lot of the literature, the term is invoked because a special estimating procedure has to be used. This is usually the case when one quantity depends on the other and vice versa so that an analytic solution to the problem is intractable. How exactly joint estimation is done depends entirely on the problem.
One method that pops up often for "joint modeling" or joint estimation is the EM-algorithm. EM stands for expectation - maximization. By alternating these steps, the E-step fills in the missing data that otherwise depend on component A, and the M-step finds optimal estimates for component B. By iterating the E and M steps, you can find a maximum likelihood estimate of A and B, thus jointly estimate these things.
$endgroup$
1
$begingroup$
Can you give an example of where we're not estimating the mean and standard deviation of one variable? What sort of algorithm is used then?
$endgroup$
– smci
Oct 3 at 17:29
$begingroup$
@smci linear mixed modeling jointly estimates the fixed and random components.
$endgroup$
– AdamO
Oct 4 at 21:59
$begingroup$
thanks, I mean, please edit that (and any other examples) into your answer. Is the algorithm totally different to EM? (How does it manage to estimate both components simultaneously? Does it guarantee convergence? etc.)
$endgroup$
– smci
Oct 4 at 22:10
$begingroup$
@smci I disagree. a) That's not the OP's question. b) There are infinite "other examples" c) what is LME's algorithm and how is it different from EM is another question.
$endgroup$
– AdamO
Oct 7 at 15:32
$begingroup$
it helps to illustrate the answer with examples. And that makes for a betetr answer, hence is part of what was asked.
$endgroup$
– smci
Oct 7 at 21:30
add a comment
|
$begingroup$
Joint estimation is, simply, jointly estimating two (or more) things at the same time. It can be as simple as estimating the mean and standard deviation from a sample.
In a lot of the literature, the term is invoked because a special estimating procedure has to be used. This is usually the case when one quantity depends on the other and vice versa so that an analytic solution to the problem is intractable. How exactly joint estimation is done depends entirely on the problem.
One method that pops up often for "joint modeling" or joint estimation is the EM-algorithm. EM stands for expectation - maximization. By alternating these steps, the E-step fills in the missing data that otherwise depend on component A, and the M-step finds optimal estimates for component B. By iterating the E and M steps, you can find a maximum likelihood estimate of A and B, thus jointly estimate these things.
$endgroup$
Joint estimation is, simply, jointly estimating two (or more) things at the same time. It can be as simple as estimating the mean and standard deviation from a sample.
In a lot of the literature, the term is invoked because a special estimating procedure has to be used. This is usually the case when one quantity depends on the other and vice versa so that an analytic solution to the problem is intractable. How exactly joint estimation is done depends entirely on the problem.
One method that pops up often for "joint modeling" or joint estimation is the EM-algorithm. EM stands for expectation - maximization. By alternating these steps, the E-step fills in the missing data that otherwise depend on component A, and the M-step finds optimal estimates for component B. By iterating the E and M steps, you can find a maximum likelihood estimate of A and B, thus jointly estimate these things.
edited Oct 2 at 19:27
answered Oct 2 at 17:50
AdamOAdamO
41.4k2 gold badges75 silver badges162 bronze badges
41.4k2 gold badges75 silver badges162 bronze badges
1
$begingroup$
Can you give an example of where we're not estimating the mean and standard deviation of one variable? What sort of algorithm is used then?
$endgroup$
– smci
Oct 3 at 17:29
$begingroup$
@smci linear mixed modeling jointly estimates the fixed and random components.
$endgroup$
– AdamO
Oct 4 at 21:59
$begingroup$
thanks, I mean, please edit that (and any other examples) into your answer. Is the algorithm totally different to EM? (How does it manage to estimate both components simultaneously? Does it guarantee convergence? etc.)
$endgroup$
– smci
Oct 4 at 22:10
$begingroup$
@smci I disagree. a) That's not the OP's question. b) There are infinite "other examples" c) what is LME's algorithm and how is it different from EM is another question.
$endgroup$
– AdamO
Oct 7 at 15:32
$begingroup$
it helps to illustrate the answer with examples. And that makes for a betetr answer, hence is part of what was asked.
$endgroup$
– smci
Oct 7 at 21:30
add a comment
|
1
$begingroup$
Can you give an example of where we're not estimating the mean and standard deviation of one variable? What sort of algorithm is used then?
$endgroup$
– smci
Oct 3 at 17:29
$begingroup$
@smci linear mixed modeling jointly estimates the fixed and random components.
$endgroup$
– AdamO
Oct 4 at 21:59
$begingroup$
thanks, I mean, please edit that (and any other examples) into your answer. Is the algorithm totally different to EM? (How does it manage to estimate both components simultaneously? Does it guarantee convergence? etc.)
$endgroup$
– smci
Oct 4 at 22:10
$begingroup$
@smci I disagree. a) That's not the OP's question. b) There are infinite "other examples" c) what is LME's algorithm and how is it different from EM is another question.
$endgroup$
– AdamO
Oct 7 at 15:32
$begingroup$
it helps to illustrate the answer with examples. And that makes for a betetr answer, hence is part of what was asked.
$endgroup$
– smci
Oct 7 at 21:30
1
1
$begingroup$
Can you give an example of where we're not estimating the mean and standard deviation of one variable? What sort of algorithm is used then?
$endgroup$
– smci
Oct 3 at 17:29
$begingroup$
Can you give an example of where we're not estimating the mean and standard deviation of one variable? What sort of algorithm is used then?
$endgroup$
– smci
Oct 3 at 17:29
$begingroup$
@smci linear mixed modeling jointly estimates the fixed and random components.
$endgroup$
– AdamO
Oct 4 at 21:59
$begingroup$
@smci linear mixed modeling jointly estimates the fixed and random components.
$endgroup$
– AdamO
Oct 4 at 21:59
$begingroup$
thanks, I mean, please edit that (and any other examples) into your answer. Is the algorithm totally different to EM? (How does it manage to estimate both components simultaneously? Does it guarantee convergence? etc.)
$endgroup$
– smci
Oct 4 at 22:10
$begingroup$
thanks, I mean, please edit that (and any other examples) into your answer. Is the algorithm totally different to EM? (How does it manage to estimate both components simultaneously? Does it guarantee convergence? etc.)
$endgroup$
– smci
Oct 4 at 22:10
$begingroup$
@smci I disagree. a) That's not the OP's question. b) There are infinite "other examples" c) what is LME's algorithm and how is it different from EM is another question.
$endgroup$
– AdamO
Oct 7 at 15:32
$begingroup$
@smci I disagree. a) That's not the OP's question. b) There are infinite "other examples" c) what is LME's algorithm and how is it different from EM is another question.
$endgroup$
– AdamO
Oct 7 at 15:32
$begingroup$
it helps to illustrate the answer with examples. And that makes for a betetr answer, hence is part of what was asked.
$endgroup$
– smci
Oct 7 at 21:30
$begingroup$
it helps to illustrate the answer with examples. And that makes for a betetr answer, hence is part of what was asked.
$endgroup$
– smci
Oct 7 at 21:30
add a comment
|
$begingroup$
In a statistical context, the term "joint estimation" could conceivably mean one of two things:
- The simultaneous estimation of two or more scalar parameters (or equivalently, the estimation of a vector parameter with at least two elements); or
- The estimation of a single parameter pertaining to a joint (e.g., in the study of carpentry, plumbing systems, or marijuana smoking).
Of those two options, the second one is a joke, so almost certainly, joint estimation refers to simultaneously estimating two scalar parameters at once.
$endgroup$
1
$begingroup$
to be pedantic, two or more
$endgroup$
– qwr
Oct 3 at 18:23
1
$begingroup$
Pedantry accepted - edited.
$endgroup$
– Reinstate Monica
Oct 3 at 22:20
add a comment
|
$begingroup$
In a statistical context, the term "joint estimation" could conceivably mean one of two things:
- The simultaneous estimation of two or more scalar parameters (or equivalently, the estimation of a vector parameter with at least two elements); or
- The estimation of a single parameter pertaining to a joint (e.g., in the study of carpentry, plumbing systems, or marijuana smoking).
Of those two options, the second one is a joke, so almost certainly, joint estimation refers to simultaneously estimating two scalar parameters at once.
$endgroup$
1
$begingroup$
to be pedantic, two or more
$endgroup$
– qwr
Oct 3 at 18:23
1
$begingroup$
Pedantry accepted - edited.
$endgroup$
– Reinstate Monica
Oct 3 at 22:20
add a comment
|
$begingroup$
In a statistical context, the term "joint estimation" could conceivably mean one of two things:
- The simultaneous estimation of two or more scalar parameters (or equivalently, the estimation of a vector parameter with at least two elements); or
- The estimation of a single parameter pertaining to a joint (e.g., in the study of carpentry, plumbing systems, or marijuana smoking).
Of those two options, the second one is a joke, so almost certainly, joint estimation refers to simultaneously estimating two scalar parameters at once.
$endgroup$
In a statistical context, the term "joint estimation" could conceivably mean one of two things:
- The simultaneous estimation of two or more scalar parameters (or equivalently, the estimation of a vector parameter with at least two elements); or
- The estimation of a single parameter pertaining to a joint (e.g., in the study of carpentry, plumbing systems, or marijuana smoking).
Of those two options, the second one is a joke, so almost certainly, joint estimation refers to simultaneously estimating two scalar parameters at once.
edited Oct 3 at 22:19
answered Oct 2 at 19:58


Reinstate MonicaReinstate Monica
44.4k2 gold badges53 silver badges181 bronze badges
44.4k2 gold badges53 silver badges181 bronze badges
1
$begingroup$
to be pedantic, two or more
$endgroup$
– qwr
Oct 3 at 18:23
1
$begingroup$
Pedantry accepted - edited.
$endgroup$
– Reinstate Monica
Oct 3 at 22:20
add a comment
|
1
$begingroup$
to be pedantic, two or more
$endgroup$
– qwr
Oct 3 at 18:23
1
$begingroup$
Pedantry accepted - edited.
$endgroup$
– Reinstate Monica
Oct 3 at 22:20
1
1
$begingroup$
to be pedantic, two or more
$endgroup$
– qwr
Oct 3 at 18:23
$begingroup$
to be pedantic, two or more
$endgroup$
– qwr
Oct 3 at 18:23
1
1
$begingroup$
Pedantry accepted - edited.
$endgroup$
– Reinstate Monica
Oct 3 at 22:20
$begingroup$
Pedantry accepted - edited.
$endgroup$
– Reinstate Monica
Oct 3 at 22:20
add a comment
|
$begingroup$
Joint estimation is using data to estimate two or more parameters at the same time. Separate estimation evaluates each parameter one at a time.
Estimation is the result of some form of optimization process. Because of this, there do not exist unique estimation solutions in statistics. If you change your goal, then you change what is optimal. When you first learn things such as regression, no one tells you why you are doing what you are doing. The goal of the instructor is to give you a degree of basic functionality using methods that work in a wide range of circumstances. At the beginning, you are not learning about regression. Instead, you are learning one or two regression methods that are widely applicable in a wide range of circumstances.
The fact you are looking for solutions that solve a hidden goal makes it a bit difficult to understand.
In the context of regression, imagine the following algebraic expression is true $$z=beta_xx+beta_yy+alpha$$. A truism in statistics is the more information that you have, the better off you are. Let us assume that you need to determine what values for $z$ will happen when you see $(x,y)$. The problem is that you do not know the true values for $beta_x,beta_y,alpha$. You have a large, complete data set of $x,y,z$.
In separate estimation, you would estimate one parameter at a time. In joint estimation, you would estimate all of them at once.
As a rule of thumb, joint estimation is more accurate than a separate estimate with a large complete data set. There is one general exception to that. Imagine you have a large set of $x$ and $z$ but a small set of $y$. Imagine most of your $y$ values are missing.
In many estimation routines, you would delete the missing $x$s and $z$s and reduce down the set you are working from until all sets are complete. If you have deleted enough data, it can be more accurate to use the large number of $x$s and $z$s separately to estimate $z=beta_xx+alpha$ and $z=beta_yy+alpha$ than together.
Now as to how it is done. All estimation, excluding a few exceptional cases, uses calculus to find an estimator that minimizes some form of loss or some type of risk. The concern is that you will be unlucky in choosing your sample. Unfortunately, there is an infinite number of loss functions. There is also an infinite number of risk functions.
I found several videos for you because it is a giant topic so that you can look at it in a more general form. They are from Mathematical Monk.
https://www.youtube.com/watch?v=6GhSiM0frIk
https://www.youtube.com/watch?v=5SPm4TmYTX0
https://www.youtube.com/watch?v=b1GxZdFN6cY
and
https://www.youtube.com/watch?v=WdnP1gmb8Hw.
$endgroup$
add a comment
|
$begingroup$
Joint estimation is using data to estimate two or more parameters at the same time. Separate estimation evaluates each parameter one at a time.
Estimation is the result of some form of optimization process. Because of this, there do not exist unique estimation solutions in statistics. If you change your goal, then you change what is optimal. When you first learn things such as regression, no one tells you why you are doing what you are doing. The goal of the instructor is to give you a degree of basic functionality using methods that work in a wide range of circumstances. At the beginning, you are not learning about regression. Instead, you are learning one or two regression methods that are widely applicable in a wide range of circumstances.
The fact you are looking for solutions that solve a hidden goal makes it a bit difficult to understand.
In the context of regression, imagine the following algebraic expression is true $$z=beta_xx+beta_yy+alpha$$. A truism in statistics is the more information that you have, the better off you are. Let us assume that you need to determine what values for $z$ will happen when you see $(x,y)$. The problem is that you do not know the true values for $beta_x,beta_y,alpha$. You have a large, complete data set of $x,y,z$.
In separate estimation, you would estimate one parameter at a time. In joint estimation, you would estimate all of them at once.
As a rule of thumb, joint estimation is more accurate than a separate estimate with a large complete data set. There is one general exception to that. Imagine you have a large set of $x$ and $z$ but a small set of $y$. Imagine most of your $y$ values are missing.
In many estimation routines, you would delete the missing $x$s and $z$s and reduce down the set you are working from until all sets are complete. If you have deleted enough data, it can be more accurate to use the large number of $x$s and $z$s separately to estimate $z=beta_xx+alpha$ and $z=beta_yy+alpha$ than together.
Now as to how it is done. All estimation, excluding a few exceptional cases, uses calculus to find an estimator that minimizes some form of loss or some type of risk. The concern is that you will be unlucky in choosing your sample. Unfortunately, there is an infinite number of loss functions. There is also an infinite number of risk functions.
I found several videos for you because it is a giant topic so that you can look at it in a more general form. They are from Mathematical Monk.
https://www.youtube.com/watch?v=6GhSiM0frIk
https://www.youtube.com/watch?v=5SPm4TmYTX0
https://www.youtube.com/watch?v=b1GxZdFN6cY
and
https://www.youtube.com/watch?v=WdnP1gmb8Hw.
$endgroup$
add a comment
|
$begingroup$
Joint estimation is using data to estimate two or more parameters at the same time. Separate estimation evaluates each parameter one at a time.
Estimation is the result of some form of optimization process. Because of this, there do not exist unique estimation solutions in statistics. If you change your goal, then you change what is optimal. When you first learn things such as regression, no one tells you why you are doing what you are doing. The goal of the instructor is to give you a degree of basic functionality using methods that work in a wide range of circumstances. At the beginning, you are not learning about regression. Instead, you are learning one or two regression methods that are widely applicable in a wide range of circumstances.
The fact you are looking for solutions that solve a hidden goal makes it a bit difficult to understand.
In the context of regression, imagine the following algebraic expression is true $$z=beta_xx+beta_yy+alpha$$. A truism in statistics is the more information that you have, the better off you are. Let us assume that you need to determine what values for $z$ will happen when you see $(x,y)$. The problem is that you do not know the true values for $beta_x,beta_y,alpha$. You have a large, complete data set of $x,y,z$.
In separate estimation, you would estimate one parameter at a time. In joint estimation, you would estimate all of them at once.
As a rule of thumb, joint estimation is more accurate than a separate estimate with a large complete data set. There is one general exception to that. Imagine you have a large set of $x$ and $z$ but a small set of $y$. Imagine most of your $y$ values are missing.
In many estimation routines, you would delete the missing $x$s and $z$s and reduce down the set you are working from until all sets are complete. If you have deleted enough data, it can be more accurate to use the large number of $x$s and $z$s separately to estimate $z=beta_xx+alpha$ and $z=beta_yy+alpha$ than together.
Now as to how it is done. All estimation, excluding a few exceptional cases, uses calculus to find an estimator that minimizes some form of loss or some type of risk. The concern is that you will be unlucky in choosing your sample. Unfortunately, there is an infinite number of loss functions. There is also an infinite number of risk functions.
I found several videos for you because it is a giant topic so that you can look at it in a more general form. They are from Mathematical Monk.
https://www.youtube.com/watch?v=6GhSiM0frIk
https://www.youtube.com/watch?v=5SPm4TmYTX0
https://www.youtube.com/watch?v=b1GxZdFN6cY
and
https://www.youtube.com/watch?v=WdnP1gmb8Hw.
$endgroup$
Joint estimation is using data to estimate two or more parameters at the same time. Separate estimation evaluates each parameter one at a time.
Estimation is the result of some form of optimization process. Because of this, there do not exist unique estimation solutions in statistics. If you change your goal, then you change what is optimal. When you first learn things such as regression, no one tells you why you are doing what you are doing. The goal of the instructor is to give you a degree of basic functionality using methods that work in a wide range of circumstances. At the beginning, you are not learning about regression. Instead, you are learning one or two regression methods that are widely applicable in a wide range of circumstances.
The fact you are looking for solutions that solve a hidden goal makes it a bit difficult to understand.
In the context of regression, imagine the following algebraic expression is true $$z=beta_xx+beta_yy+alpha$$. A truism in statistics is the more information that you have, the better off you are. Let us assume that you need to determine what values for $z$ will happen when you see $(x,y)$. The problem is that you do not know the true values for $beta_x,beta_y,alpha$. You have a large, complete data set of $x,y,z$.
In separate estimation, you would estimate one parameter at a time. In joint estimation, you would estimate all of them at once.
As a rule of thumb, joint estimation is more accurate than a separate estimate with a large complete data set. There is one general exception to that. Imagine you have a large set of $x$ and $z$ but a small set of $y$. Imagine most of your $y$ values are missing.
In many estimation routines, you would delete the missing $x$s and $z$s and reduce down the set you are working from until all sets are complete. If you have deleted enough data, it can be more accurate to use the large number of $x$s and $z$s separately to estimate $z=beta_xx+alpha$ and $z=beta_yy+alpha$ than together.
Now as to how it is done. All estimation, excluding a few exceptional cases, uses calculus to find an estimator that minimizes some form of loss or some type of risk. The concern is that you will be unlucky in choosing your sample. Unfortunately, there is an infinite number of loss functions. There is also an infinite number of risk functions.
I found several videos for you because it is a giant topic so that you can look at it in a more general form. They are from Mathematical Monk.
https://www.youtube.com/watch?v=6GhSiM0frIk
https://www.youtube.com/watch?v=5SPm4TmYTX0
https://www.youtube.com/watch?v=b1GxZdFN6cY
and
https://www.youtube.com/watch?v=WdnP1gmb8Hw.
answered Oct 2 at 18:47
Dave HarrisDave Harris
4,6517 silver badges18 bronze badges
4,6517 silver badges18 bronze badges
add a comment
|
add a comment
|
Thanks for contributing an answer to Cross Validated!
- Please be sure to answer the question. Provide details and share your research!
But avoid …
- Asking for help, clarification, or responding to other answers.
- Making statements based on opinion; back them up with references or personal experience.
Use MathJax to format equations. MathJax reference.
To learn more, see our tips on writing great answers.
Sign up or log in
StackExchange.ready(function ()
StackExchange.helpers.onClickDraftSave('#login-link');
);
Sign up using Google
Sign up using Facebook
Sign up using Email and Password
Post as a guest
Required, but never shown
StackExchange.ready(
function ()
StackExchange.openid.initPostLogin('.new-post-login', 'https%3a%2f%2fstats.stackexchange.com%2fquestions%2f429712%2fwhat-is-joint-estimation%23new-answer', 'question_page');
);
Post as a guest
Required, but never shown
Sign up or log in
StackExchange.ready(function ()
StackExchange.helpers.onClickDraftSave('#login-link');
);
Sign up using Google
Sign up using Facebook
Sign up using Email and Password
Post as a guest
Required, but never shown
Sign up or log in
StackExchange.ready(function ()
StackExchange.helpers.onClickDraftSave('#login-link');
);
Sign up using Google
Sign up using Facebook
Sign up using Email and Password
Post as a guest
Required, but never shown
Sign up or log in
StackExchange.ready(function ()
StackExchange.helpers.onClickDraftSave('#login-link');
);
Sign up using Google
Sign up using Facebook
Sign up using Email and Password
Sign up using Google
Sign up using Facebook
Sign up using Email and Password
Post as a guest
Required, but never shown
Required, but never shown
Required, but never shown
Required, but never shown
Required, but never shown
Required, but never shown
Required, but never shown
Required, but never shown
Required, but never shown
PA1mZnq7 qTWQLl8N9cbVEDYjoNyPZzuH
1
$begingroup$
Thank you very much for all the helpful answers and the effort you put to clarifying this to me!
$endgroup$
– Lost in regression
Oct 3 at 15:16